Course Overview
This course aims at providing the student with an overview of python and its libraries, with a focus on industrial application. One will gain hands-on experience by working on refinery-specific use cases and will get the opportunity to build models from scratch up to their final visualization.
Topics Covered
- What is Machine Learning
- Creating a Digital Twin
- Creating a Machine Learning Model
- Feature Engineering
Course Curriculam
Python
Numpy and Pandas
Feature Engineering
Projects you will build
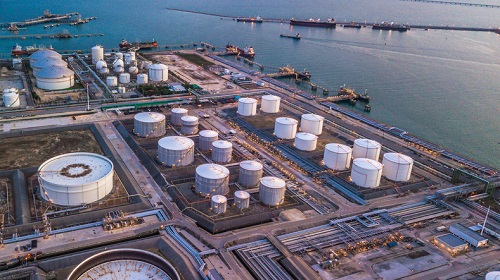
Artificial Intelligence
AST Bottom Plate Monitoring
Corrosion Monitoring, Predictive Maintenance